Outsourcing to Poland
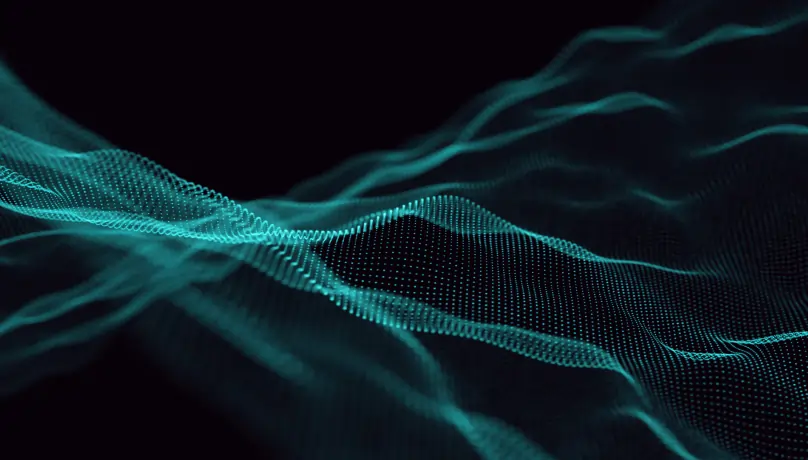
How to choose the best outsourcing software development company in Poland?
Jul 1st 25 - by Devico Team
Learn how to choose the best software development outsourcing company in Poland that delivers quality and value.
Hire
Hire by role
Hire Front-end developers
Hire Back-end developers
Hire Full-stack developers
Hire Android developers
Hire iOS developers
Hire Mobile developers
Hire AI engineers
Hire ML engineers
Hire Automation QA engineers
Hire Blockchain developers
Hire Data engineers
Hire Cloud engineers
Hire by skill
Hire JavaScript developers
Hire TypeScript developers
Hire Ruby on Rails developers
Hire React Native developers
Hire Flutter developers
Hire Golang developers
Hire React.js developers
Hire Python developers
Hire PHP developers
Hire .NET developers
Hire Java developers
Hire Laravel developers
Data engineering
July 01, 2024 - by Devico Team
“He who owns the information owns the world”. By saying this phrase, Nathan Rothschild could not have imagined how relevant it would be in the age of digital technologies.
Today, absolutely everything is turned into data, every swipe on the screen, a click on a website, a view of any page, a picture, a line in a text. These simple, ordinary, everyday things transform industries, and lead companies to leadership and breakthrough solutions but only if they are used correctly.
Most modern decision-making tools are built on the in-depth analysis of information that is used for improving services, clarifying the needs and requirements of customers, identifying potential threats and risks, forecasting, and so on. According to an MIT report, only 13% of organizations are currently succeeding in their data strategy. Therefore, the real key to success is proper data management that includes a comprehensive approach to collecting and processing all the data across the organization.
So, let's find out together what data management is, why it is crucial now, and how it can help your business.
Data management stands for a comprehensive process of collecting, processing, analyzing, and using information across the organization. The main task of the data management system is to increase the efficiency and value of the information, hence, it must be secure, effective, and comply with the regulations.
As data becomes essential to organizational success and the volume and complexity of data increases, data management is now a vital component of the growth strategies of small and large companies. Nevertheless, a Harvard Business Review study states that 60% to 73% of all data in an enterprise is not used for analytics. This is where data management systems come in handy, as they allow to unlock the full potential and power of the information:
With effective data management, startups and small businesses can grow faster, be more agile, make better decisions, and gain competitive advantages to become market leaders.
Large companies and corporations can solve a few common issues managing data: ensuring data integrity and accuracy across departments, maintaining a unified strategy, and operational efficiency.
A sound and robust data management process enables companies to leverage big data and other technologies such as cloud computing, artificial intelligence, and machine learning for more sophisticated analytics and to meet stringent compliance requirements.
Effective data management increases productivity, improves customer satisfaction, reduces risks associated with data leakage, and leads to innovation and improved services.
In a relatively short time, data management, which began with the storage of physical (paper) files in back offices, has turned into one of the front lines of business planning and strategizing. Much of this is due to the digitization of processes that originally transferred files to digital storage, such as warehouses and servers, and now transfer them to cloud storage for increased efficiency. Over time, not only the ways of storing and using data have changed, but also the logic of their processing and further use for the company's profitability. By applying modern data management technology, companies unlock almost limitless capabilities for growth and development.
From general points to specific ones: management of data can handle lots of issues and benefit the company in several crucial areas, enhancing business operations and performance. This is how an efficient and robust management data strategy can impact your company.
With access to more complete information, managers can make better and more informed decisions based on high-quality data. Having a complete picture of business performance, organizations can anticipate potential problems and risks and take preventive measures. Access to large volumes of high-quality, comprehensive data from a variety of sources is key to using all machine learning (ML) and artificial intelligence (AI) algorithms capabilities. It allows you to automate and scale decision-making, predict future events, and react in real time.
For example, Coca-Cola was one of the first companies outside the IT market that adopt big data and AI to enhance their performance. By combining these technologies, they were able to track mentions of their brand on social media and determine the most effective way to promote their products. By making a bold data-driven decision, they targeted advertising in a new way. This resulted in four times the likelihood of an ad being clicked compared to other targeted advertising methods.
Quick access to up-to-date data allows employees to work more productively and efficiently. No more time spent searching for different data sets from other departments or systems or manually entering information from other sources. This means they can focus on using data to do their jobs more effectively, contributing to overall competitiveness.
Automation of most processes solves several tasks at once: reduce errors, cut labor costs, and fasten decision-making. Constant access to cleaned, consistent and reliable data in real-time allows you to quickly adapt to the changing market. For example, to research users' opinions to introduce new options and products, or to adjust marketing strategies for their rapid and effective promotion, allocating resources for maximum benefit.
As it is clear from the data management definition, it allows the collection and analysis of large amounts of data, which is essential for customer-centric business. Proper data management provides businesses with comprehensive information on customer journeys, demands, requirements, and preferences. Based on this information, they can tailor their products and services for better personalization. By applying data management for forecasting and identifying new trends, companies can proactively adjust their strategies to improve service quality.
For instance, companies like Amazon and Netflix use customer data for personalized recommendations based on previous interactions, significantly enhancing user experience and satisfaction.
Speed can play an important role in advancing a business, especially when it comes to introducing new options or products. Having access to a large amount of information, companies can be the first to predict market trends, develop the necessary solutions, and provide them to their customers.
It also opens the door for innovations that are created based on the received data. In this way, businesses will get a significant advantage and can bypass their competitors, gaining better and higher positions.
Most companies today collect, store, and process users' personal information. The protection of this data must meet strict regulatory standards such as GDPR and CCPA. And this, as a result, requires companies to implement the necessary data safety and encryption protocols. With clear processes and audit trails, a company can always be aware and approve who has access to data sets and for what.
An effective data management strategy allows you to map all data, ensure its protection, and use it only for certain purposes. This ensures regulatory compliance and protects the brand's reputation.
Today, any information that enters the organization or is collected by it through open channels goes through several stages or the so-called lifecycle. Data management processes include several main stages that may vary depending on the architecture and the company’s internal policy of working with information.
The first stage of data management is literally collecting data from various sources. Several main methods of data collection can be used by companies for various purposes. For example, to gather users' or customers’ opinions, surveys, marketing research, and user journey info are most often used. Have you seen popup messages about cookies when you visit different websites? This is one of the data collection methods. Here are some more non-obvious ways to get access to data:
Reports: both internal and external. They allow you to systematize data from various categories and see patterns more quickly. For example, government reports are crucial to the financial and healthcare industry.
Sensors are used in many areas, such as manufacturing, logistics, commerce, agriculture, and healthcare. They provide real-time data for monitoring and analysis.
Customer forms are widely used in e-commerce, customer support, and registration sites. These forms collect data directly from users.
Social networks are a rich source for analyzing sentiments, trends, and customer feedback. Posts, likes, comments, and shares are tracked.
However, when collecting a large amount of data for in-depth analysis and improved decision-making, it is necessary to consider the quality of the data. There are often issues with the verification and validation of the obtained information, its reliability, and truthfulness. You might need additional software or tools to ensure high data quality before proceeding to analyses.
So, you have collected a lot of data and now these terabytes of information must be stored correctly and efficiently. In addition to choosing between physical storage and cloud solutions, you must also select the format and methods; keep in mind the availability, systematization, and flexibility of the data. Depending on your needs, the choice of storage solution affects the way data is managed and used:
Databases are structured to support operations and query processing. They lack flexibility, but the risk of errors is minimized, making them perfect for transactional operations.
Data warehouses are optimized for analysis and reporting. They provide a central repository of historical and current data in a convenient format.
Data lakes are designed to store massive amounts of raw data in a native format. Suitable for storing different types of data from various sources, offering flexibility for data professionals and analysts.
Among the main disadvantages of most data storage methods is their cost, because the more information you store, the more your costs increase.
One of the challenges of storing a large amount of data is ensuring its security and protection against third-party interference. In recent years, the number of cyber-attacks has increased significantly, which requires businesses to use additional and reliable means of securing sensitive information.
Powerful firewalls provide additional security for your data. Regular updates to security protocols allow you to avoid gaps in data protection.
Encryption using the latest methods like blockchain or other technologies is an available method of storing data integrity.
Access control to information allows you to limit the number of users and reduce the risk of information leakage and falling into third hands. This includes double authorization, constant updating of reliable passwords, and more.
If you use public cloud or hybrid storage to store your company's sensitive information, you should be absolutely sure of your provider or implement additional methods.
At this stage of data management, data from different sources is combined into a single repository, such as a data warehouse or data lake, breaking down data silos. This allows to get a unified view and makes it easier for organizations to analyze and obtain valuable information. Data integration also simplifies the decision-making chain for organizations by enabling better reporting and predictive analytics.
The most common data integration technique is extract, transform, and load (ETL). In simple words, it takes data from source systems, transforms it into a consistent format, and then loads it into a data warehouse or other target system. However, data integration platforms now also support many other integration methods.
Data integration can be challenging due to differences in data formats, duplication, and sheer volume of data. Effective integration requires robust methodologies and technologies for data synchronization, deduplication, and consolidation.
At this stage of the data management processes, the most interesting things happen: most of the insights and breakthrough solutions become obvious after a deep analysis of the data. Using various techniques, specialists receive valuable information that enhances decision-making and ensures competitive advantage. Before analysis, the data is usually additionally cleaned and checked, as a rule, flexible methods and modern tools are used for this.
ML algorithms. Thanks to artificial intelligence and machine learning implementation, the analysis of big data takes place with the help of the latest algorithms, which allows you to systematize the data in a convenient form of a categorized report in a short time.
Business intelligence (BI) tools. After preparing the data, business intelligence tools are used to create reports and queries about them.
Data visualization tools. Data visualization software allows users to transform tabular data into maps, dashboards, and other graphical forms. This makes it more attractive and understandable for users.
In fact, data management does not end with their analysis, data can be sent to storage or deleted depending on their importance and relevance.
Learn top strategies for improving your data engineering workflows
Creating a strategy to manage data involves the application of additional tools or data management components that will be useful to all companies regardless of their size and industry. In creating a data management system, it is necessary to consider not only the current volumes but also to foresee their gradual growth and increase in capacity for their processing. That is, when choosing tools, keep in mind that over time they may not be enough for your business, always leave room for updates and growth.
At the heart of data management are database management systems (DBMS) — programs designed to create and manage databases for data storage. With DBMS, users can define, create, retrieve, update, and manipulate data in a database, ensuring day-to-day operation and data availability. DBMS have different types, in particular:
Relational databases use a structured format to store data in tables with predefined relationships between them. They are used to process complex queries and maintain data integrity.
In-memory databases store data in the server's main memory. This ensures faster data access and processing.
NoSQL databases were designed to work with the Internet and the cloud as support systems. Widely used for real-time analytics, such as the Internet of Things, the customer experience, and social media feeds.
Data warehouses are centralized storage and data management systems for collecting data from various sources and further analysis. As a single repository of highly structured and unified historical data, it is most often used by large companies and corporations. It is designed to support additional tools and seamless integration with BI, data analysis, AI, and machine learning algorithms to provide informed decision-making and improve organizational performance. In addition, data warehouses allow you to store already cleaned information, regardless of whether you collect validated data immediately or use an ETL pipeline.
Since the information is already structured and stored in the proper order, you get not only faster access to it, but also significantly boost the speed of data processing and, as a result, enhance efficiency in general. In addition, it is a flexible and scalable storage solution that can be easily used for further growth and an increase in data volumes. If you use a reliable service provider, you also get additional data security measures.
Data lakes are systems that store raw, unstructured, and semi-structured data at scale. They allow you to store huge amounts of data in a native format, offering high flexibility for conducting exploratory analytics.
Data lakes typically use highly scalable data storage tech that can scale as the volume of data increases. This ensures that data lakes can handle petabytes of data without significant performance degradation.
It is also a cost-effective solution, as you reduce expenses for data preparation (cleaning and transformation into the necessary format), and shift to affordable cloud storage. Data lakes allow you to modernize your digital infrastructure, open access to the use of new technologies, and give you enough freedom to work with data without compromising security.
Master Data Management (MDM) is responsible for data consistency and how data is created, shared, updated, and used. In simple words, MDM provides context to data from the database, making it easily understandable and manageable.
Master data management software consolidates all your information sources into a single view. You can gain greater visibility with these solutions that ensure your data is accurate and actionable.
MDM helps avoid data redundancy and conflicts and ensures that all departments are working with the same version of data, increasing accuracy and consistency across the company. Consistent master data enables better customer relationship management by providing a single view of customers across all touchpoints.
When building data management processes in your company, consider not only the basic needs but also the features that may require certain software and service customizations and specifications.
Now that we've answered the ‘how does data management work’ question, let’s explore its future. First, always look ahead to new ways of data management meaning you have to adopt new methods, tech, and strategies before your potential competitors. Here are some ideas to consider.
Data fabric is an architectural concept that collects data from various sources and combines it into a single useful unit. The data fabric uses continuous analytics of existing, accessible, and inferred metadata to support the development, deployment, and use of integrated and reusable data across all environments. Access to the received data is available through one of the centralized platforms: database warehouse or lake. This solution allows to effectively manage data without changing current systems, eliminate clutter, improve scalability, and optimize data management. It is predicted that the global data fabric market is expected to expand at a compound annual growth rate (CAGR) of 21.2% by 2030.
Cloud data management solutions are becoming increasingly popular because they offer improved scalability, flexibility, and cost-effectiveness. Gartner claims that by 2025, more than 85% of organizations will adopt a cloud-first approach. Cloud storages are already adapted for reliable and seamless data integration platforms, such as cloud data warehouses and data lakes. They provide companies with a centralized and scalable solution to manage their data, enabling faster and more efficient processing and reducing costs. Among the recent use cases are hybrid and multi-cloud environments. This allows for a more stable and flexible data integration environment.
Augmented data management uses artificial intelligence (AI) and machine learning (ML) technologies to automate the processes involved in managing data. Most often, AI is used for data processing, which allows companies to reduce errors, increase accuracy, and make more informed decisions. AI is also used to improve data security, data synchronization, and migration. By bringing machine learning algorithms to create visual representations of data, and combining them with AI and automation of basic processes, you can discover hidden insights and patterns. According to Statista, AI and machine learning ML already play a key role in automation across industries, with 80% of respondents having deployed or adopted these technologies.
Also, ‘real-time’ can become a buzzword for data management shortly. A large amount of data is collected from IoT sensors, mobile devices, and social networks. It is this information that allows companies to be one step ahead of the competitors, and therefore the introduction of tools and platforms capable of working with real-time data will be key to success.
So, we hope that considering the information provided above, you will not underestimate the importance of data management for your business. Correct, appropriate, and timely use of the insights obtained from the processing of the collected information will allow you to bypass your competitors and turn into a real innovator in the industry.
Use modern tools and platforms for data storage, processing and analysis. By increasing the quality of the data you work with, you can make more informed decisions, improve operational performance and efficiency, and enhance customer service. Apply a smart and complex approach while building your own data management strategy that includes components, tools, and technologies specialized for your requirements and needs.
Outsourcing to Poland
Jul 1st 25 - by Devico Team
Learn how to choose the best software development outsourcing company in Poland that delivers quality and value.
Outsourcing to Poland
Jun 24th 25 - by Devico Team
Thinking of outsourcing to Poland? A complete guide that covers hourly rates, tech talent, legal setup, and more.
Outsourcing to Poland
Jun 17th 25 - by Devico Team
Looking to outsource software development? See how Ukraine and Poland compare on price, performance, and potential.