Outsourcing
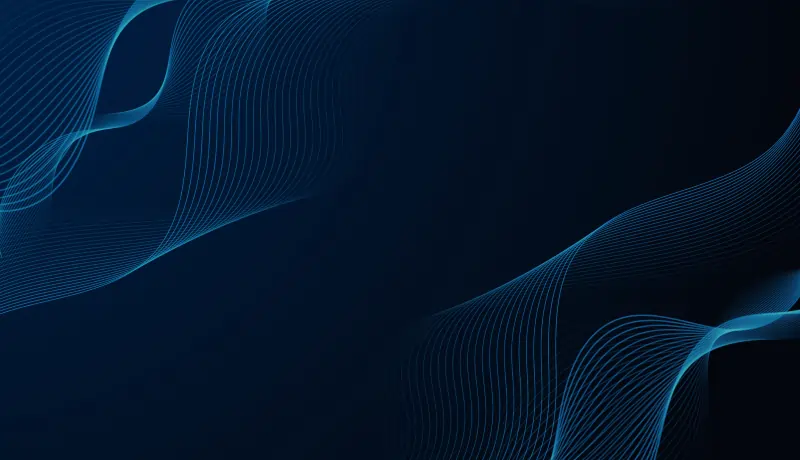
5 signs you need to outsource your software development
Apr 1st 25 - by Devico Team
Learn about the main signs that it is the right time to outsource software development to help (or even to save) your company.
Data engineering
July 10, 2024 - by Devico Team
180+ zettabytes of data. That’s exactly how much we’ll consume and generate data by 2025, according to Statista. Under such a light, data engineers seem to capture the tech world.
Simply because they are navigating a landscape where the volume, variety, and velocity of data set new benchmarks. This relentless growth is transforming modern data engineering, pushing professionals to innovate and adapt continuously to use this vast data potential effectively.
2024 has passed its halfway point, and we can definitely list several key trends and predictions that are shaping the future of data engineering (DE). Like real-time data processing, the integration of artificial intelligence (AI) in data architecture, the growing emphasis on data governance and security, and the expansion of cloud-native technologies.
Some pathos to drum up your enthusiasm: each of these trends holds the power to revolutionize data engineering practices and workflows, and if you are not running fast enough (not keeping up with these trends), you risk falling behind.
Without any further ado, dive into this brief overview and make sure you keep your hand on the industry’s pulse.
Forecasting engineering industry trends is a little bit frivolous thing since the industry itself got an unbelievable acceleration in recent years. However, we can definitely zero in on some constant patterns.
One of them is an Artificial Intelligence (AI) influence, obviously. While the complete replacement of data engineering jobs by AI is a concern, the reality is more nuanced. In 2017, McKinsey Global Institute found that while 800 million jobs could be lost to automation by 2030, an even greater number (up to 950 million) could be created.
So, the trend is obvious — AI-powered tools augment human capabilities rather than replace them entirely. McKinsey also found the most popular ways of AI usage in 2023. As of now, there are marketing tasks (and content creation in particular) and organizing tasks like summarizing text documents or gaining main insights into a certain topic.
But let’s turn back to trends in engineering. The AI’s ability to automate repetitive tasks that consume valuable time streamlines processes across the entire data lifecycle:
Data ingestion: AI can efficiently pull data from diverse sources, automatically identifying and configuring connections to databases, APIs, and cloud storage.
Data transformation: AI can automatically identify and apply the necessary transformations to format the data consistently. Recall how often you needed to conduct data type conversion, handle missing values, and normalize.
Data cleansing: A tedious process of removing errors and inconsistencies, data cleansing can be significantly improved with AI-powered anomaly detection and correction techniques. You can train a certain AI model on past data patterns to identify and rectify inconsistencies.
Data lineage management: Artificial Intelligence can play a crucial role in the process of tracking the origin and flow of data. If we are talking about trends in engineering, chances are more and more data engineers will gain a deeper understanding of how data is used throughout the organization through machine learning algorithms. This way, companies will enhance their data quality and compliance.
Several AI-powered tools are already making waves in the data engineering world. Companies like Databricks offer tools like Delta Lake that leverage machine learning for data quality and reliability. Trifacta Wrangler, another player in the field, uses machine learning to automate data-wrangling tasks like schema detection and data cleaning.
It seems like everyone's catching the FinOps fever lately, especially when it comes to data trends. The chant from across the boardrooms is loud and clear: "Show me the money!" Businesses are all about doing more with less. It's not just about cutting costs, though; adding "business value" is the new buzz.
Data engineering squads are stepping up their game, getting savvy with the financial side of data ops. They're finding ways to optimize costs and milk their tools and infrastructure for all they're worth in terms of efficiency gains. But here's a twist: FinOps isn't just a penny-pinching exercise. It's also about using data smartly to open up new revenue streams.
Key points:
FinOps is becoming a big deal in the data world. It pushes teams to get better at managing costs and boosts efficiency.
FinOps is equally about leveraging data to pump up revenue and saving “greens”.
AI augmentation benefits can be listed for several minutes, literally. But in a nutshell, it helps with repetitive tasks and allows data engineers to focus on more strategic initiatives like data modeling, pipeline optimization, and building data-driven solutions.
As a consequence, you get increased efficiency and faster data pipelines. Additionally, AI can significantly reduce human error in data processing — more reliable and trustworthy data at the end of the day.
Cloud technologies totally transformed the approach to data management, and hence, business operations. Look at one of the most famous examples — Netflix's transition to AWS. The main idea is that scalability and global reach are achievable with cloud technologies. This principal shift allowed Netflix to complete their business shift – from CD rental to streaming broadcasting – alongside effectively managing increased demand and expanding its service worldwide. This is not to mention new tech possibilities that opened to them: smooth visual effects (VFX) usage, cross-team collaboration in a single digital space, etc.
Scalability: Cloud-native systems can dynamically scale resources based on real-time demand, which is crucial for handling varying data loads without substantial initial expenditure.
Elasticity: This feature ensures that cloud services can maintain performance levels despite fluctuating workloads, which helps to control operational costs.
Cost-effectiveness: With cloud-native architectures, companies pay only for the resources they use, which optimizes spending and reduces waste.
The evolution of managed services and serverless computing has greatly simplified data engineering tasks. Services like Amazon Redshift and Google BigQuery manage the heavy lifting of server upkeep, while data engineers zero in on insights. Serverless computing models – cloud provider manages server operations – offer an efficient way to deploy applications that scale automatically with use. This way, companies can drastically enhance their operational efficiency.
While the benefits are substantial, the transition to cloud-native architectures brings challenges, particularly in data security and governance. Recall the 2019 Capital One data breach, where sensitive information of nearly 100 million individuals in the US and 6 million in Canada were compromised due to misconfigured web application firewalls on AWS.
Key idea: Stringent security protocols and comprehensive compliance strategies help safeguard data in the cloud.
Unlike traditional centralized data management systems, where a single team handles data for the entire organization, data mesh adopts a decentralized approach. This method allocates data ownership to domain-specific teams, enabling those who are closest to the data to manage it.
Imagine you’re playing a massive video game "The World of Information". In this game, there’s a treasure hunt where you have to find and gather shiny gems called "Data". Now, the world of this game is absolutely colossal, and there are countless gems hidden in different corners. To win, you need to collect as many gems as possible, as fast as you can.
But there’s a twist: the game has special helpers called "Data Engineers". These engineers are super smart and have magical maps and tools that help them find, collect, and organize the gems way faster than anyone else.
Game evolves, and the treasure lands grow even bigger; the gems get hidden in more complex puzzles. The engineers have to level up their skills and tools to keep up. They start using even more powerful magic, like spells called "Artificial Intelligence" to automate some of their work, flying carpets named "Cloud-Native Platforms" to move faster, and secret codes called "Data Mesh" to share maps and tools with friends so everyone can find gems together more efficiently.
In centralized data management, a bottleneck often forms as a single team struggles to meet the diverse needs of the entire organization. Data mesh, in contrast, distributes this responsibility across various teams, each managing their own data as a product. This shift not only lightens the load on a central team but also tailors data handling to the specific requirements of each domain.
Context matters. Domain-specific teams manage their data as they come. That means they are the most adequate decision-makers regarding their data. Such an approach promotes a high degree of autonomy but also enforces a standardized set of protocols for data interoperability. While teams operate independently, they use common frameworks that ensure their data can seamlessly integrate with other parts of the organization.
Increased agility: Teams can quickly adapt and respond to their specific data needs without waiting for centralized decisions.
Improved data quality: Domain experts manage the data, greatly enhancing its accuracy and relevance.
Enhanced collaboration: As each team manages its data independently but follows standardized protocols for integration, there is a natural increase in collaboration and understanding across the company.
Potential drawbacks and best practices:
If you want to implement data mesh, you should embrace a new outlook and treat data as a product. Note, without proper governance, the decentralized nature can lead to inconsistencies.
Setting up a robust governance framework allows to maintain data consistency and quality across domains. Invest in training and development to ensure all teams are equipped to manage their data effectively.
Slow processing that costs an arm and a leg now has nothing to do with big data. Fast and “small” big data is one of the common points from the data engineer future. A key driver of this trend is the need for businesses to manage increasingly large volumes of data more efficiently.
IDC found that companies optimizing their big data processing become more data-driven and can reduce associated costs by up to 34%.
Learn how to drive maximum business value from big data development
This focus on efficiency is transforming how we handle massive datasets.
If you asked us to name central technologies for developing real-time data pipelines, we’d mention Apache Kafka and Amazon Kinesis. These tools allow businesses to process and analyze data as it arrives, significantly speeding up decision-making processes. For example, Uber uses Kafka to handle about 12M rides a day.
In-memory computing platforms like Apache Spark have already revolutionized big data processing by storing data in RAM instead of hard disks. This significantly reduces the time it takes to process large datasets. For instance, Alibaba uses Spark to power its real-time analytics during the Singles Day shopping event, processing up to billions of transactions per day.
Alibaba Taobao began experimenting with GraphX on Apache Spark version 0.9 and successfully transitioned to a production environment by May 2014.
The interesting thing is that GraphX is still under rapid development. While the APIs accessible to users have maintained a level of stability, the underlying technology has undergone significant changes and enhancements from version 0.8 to 1.0.
Alibaba noted that with each minor version update, they saw performance boosts of 10 - 20%, even without making any changes to their application's code.
Modern data warehousing solutions, such as Snowflake and Google BigQuery, offer flexible, scalable environments for analyzing structured and semi-structured data. These platforms support massive concurrency and are optimized for cloud environments, making them ideal for businesses scaling their data analytics capabilities.
Apache Iceberg, in particular, is carving out a niche by focusing on cost-effective, structured storage solutions. Unlike modern data warehouses and lakehouses that combine compute and storage capabilities, Iceberg excels in providing scalable storage that seamlessly integrates with a variety of data processing engines like Apache Spark, Trino, Flink, Presto, Hive, and Impala. This way, development and data engineering teams can use different tools within the company to access data concurrently without hiccups.
The momentum around Iceberg is further fueled by Databricks' move to make Delta tables metadata compatible with Iceberg, along with Snowflake's aggressive integration efforts. As lakehouse architecture cements its position as a standard for many companies, the popularity of Iceberg — and its alternatives — is set to soar, offering a flexible and efficient solution for managing data at scale.
Basically, all has already been said. Data lakes play a crucial role in storing vast amounts of structured and unstructured data. They provide a scalable and cost-effective solution for businesses that need to store petabytes of data while retaining the flexibility to use it for various types of analysis. Tools like Amazon S3 and Azure Data Lake enhance the capability of data lakes by providing extensive data management features and integration with other analytics services.
Edge computing is set to redefine data processing by enabling data to be analyzed at or near the source of generation. This technology:
reduces latency,
decreases bandwidth use,
improves response times.
All these make it crucial for real-time applications like IoT devices (security cameras, managing centers, smart house devices, etc.) and autonomous vehicles. Processing data closer to where it is created, edge computing facilitates more immediate insights and actions, which, in turn, enhances the efficiency and effectiveness of data-driven decisions.
As we’ve already understood, the future of data engineering is evolving directly in our sight. From the rise of AI-powered automation to the embrace of cloud-native solutions, these engineering trends hold immense potential for companies that want to get the most out of their data. As data meshes empower domain-specific ownership and data lakes become the go-to repository for all data types, big data processing is getting streamlined for faster performance and reduced costs.
The data engineering future belongs to professionals who can navigate this dynamic environment. Staying informed about emerging trends like real-time analytics, data governance best practices, and the adoption of open-source tools is key to remaining competitive.
Outsourcing
Apr 1st 25 - by Devico Team
Learn about the main signs that it is the right time to outsource software development to help (or even to save) your company.
Outsourcing
Mar 25th 25 - by Devico Team
Learn how outsourcing in 2025 may become the black swan for startups with its cost efficiency, talent pool, and scalability support.
Outsourcing
Mar 18th 25 - by Devico Team
Should companies still opt for software outsourcing in 2025? The answer depends on many factors and the product you want to build.