Outsourcing
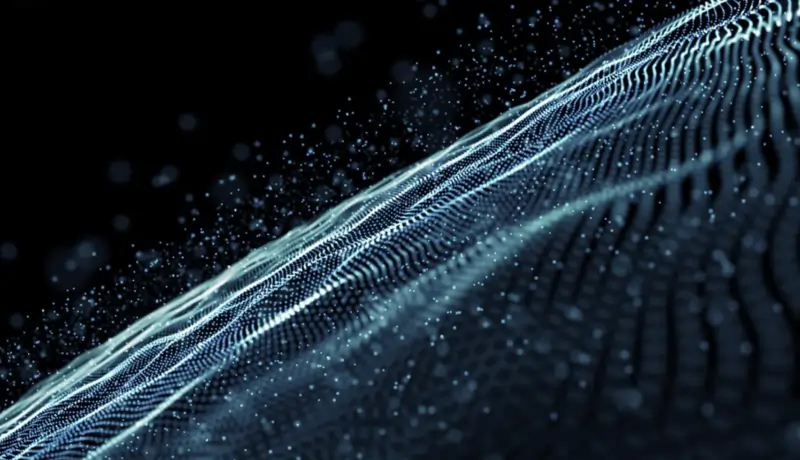
10 questions to ask your software development outsourcing vendor
Apr 16th 25 - by Devico Team
10 burning questions for software outsourcing companies to answer before you decide which one will be your partner.
digital transformation
September 14, 2024 - by Devico Team
Machine learning (ML) and artificial intelligence (AI) are driving factors in productivity, efficiency, and innovation. Embedding AI in digital transformation as well as ML efforts is no longer a nice to have; it's a need for enterprises that want to survive and grow.
The potential negative impacts of AI on the workplace are also widely believed. Pew Research surveyed American workers in 2023 and discovered that 32% of them think AI would do more harm than good to the workforce, while just 13% think the opposite. However, it should be noted that AI has the potential to greatly benefit many sectors, including digital transformation.
In this article, we provide a comprehensive and practical digital transformation checklist to help companies smoothly integrate AI/ML technology into their digital transformation projects.
When it comes to digital transformation, terms like "artificial intelligence" and "machine learning" are more than just buzzwords; they're game-changing technologies that are altering the way companies function, make choices, and engage with consumers. ML is a branch of AI that aims to equip computers with the capacity to learn and adapt autonomously from their experiences, as opposed to relying only on pre-programmed instructions. AI as a whole refers to the process of imitating human intelligence in machines.
Businesses may improve their decision-making and consumer relationships with the help of AI and ML, which in turn leads to more efficient and tailored results. As an example, Amazon saw a 35% boost in income from product suggestions alone because of their AI-powered personalized shopping experiences. American Express is another example that uses ML to drastically cut down on losses caused by fraud by analyzing customer payment data.
Improved decision-making thanks to deep insights obtained from data readiness, the identification of new business prospects via predictive analytics and trend detection, and the automation of mundane operations that free up human resources for more complicated challenges are key advantages.
According to Gartner's AI business value forecast, decision support/augmentation is the most widely used AI category because of its low initial barrier to adoption and strong business value-add. With 44% of the world's AI-derived commercial value coming from decision support and augmentation by 2030, it will have surpassed all other categories of AI projects. With AI and ML at the core of their digital transformation initiatives, businesses cannot only stay up with, but also surpass their competitors.
It is critical to analyze the foundational business structure thoroughly before diving into AI/ML deployment. Find out first what problems or possibilities your company has that AI/ML can solve very well. To better serve users all across the world, Baidu has implemented deep learning-powered voice and speech recognition, which improves user engagement by accommodating a variety of dialects and languages.
To make sure that these technologies are integrated with the overall company plans, it is important to set defined objectives, but also:
check whether data systems are prepared to back AI-driven solutions and if the current tech infrastructure can handle them;
find out what is missing from your present configuration that might prevent AI/ML integration from working well, such as out-of-date systems or inadequate data capabilities;
take into account the technology and the possibility of expert assistance when estimating the financial expenditure needed for AI/ML projects.
One example of a company that saw a significant return on investment from AI integrations is JP Morgan, which used the COIN system, which is driven by AI, to analyze legal documents. The system significantly cut down on analysis time while increasing accuracy.
It is also critical to plan for the human resources that will be required, such as hiring or training people to use AI/ML technologies properly. Just like the American Cancer Society, uses Google Cloud ML Engine to improve the detection accuracy of breast cancer. It significantly enhances patient care and integrating these preparatory steps will lay a solid foundation for successful AI/ML implementation, driving significant improvements in efficiency and innovation.
Enterprises must adhere to a systematic strategy when they integrate AI/ML into their operations. This will guarantee a smooth and successful deployment.
1. Criteria for selecting AI/ML tools and platforms
The key to a successful AI/ML deployment is picking the correct platforms and tools. Think about systems with demonstrated efficiency improvements, such as Azure Machine Learning, which aided AIMMO in improving productivity and cutting costs with its all-encompassing MLOps process. To accommodate the many steps involved in creating and deploying AI models, the platform should include a comprehensive set of features.
2. Flexibility and scalability are crucial
Make sure the AI/ML system you choose can grow with your company and adapt to new demands. By using AWS SageMaker for agricultural pest detection, Bayer AG has shown how the platform can handle scalability, react to real-time data inputs, and manage variable demand—all while dramatically decreasing costs.
3. Data management best practices
Data management is the foundation of every AI/ML project. Strong procedures for data gathering, storage, and processing must be put in place. Data integrity and usability are preserved throughout the data lifetime when an MLOps pipeline is established, as is the case with AIMMO. This pipeline facilitates efficient data flow from collection to model deployment.
4. Importance of data quality and security
Data security and quality are of the utmost importance when training models to be dependable. Protect sensitive information by implementing stringent data quality control procedures and by making sure you comply with data protection laws. Building successful and secure models requires high-quality data, as shown by Microsoft's AI-powered accessibility solutions.
5. Required skill sets (Data Scientists, ML Engineers, etc.)
Bring on board people with data science and ML experience, or train them yourself. An example of the requirement for trained experts who can use AI technologies to enhance medical diagnostics is the American Cancer Society's use of Google Cloud ML Engine for cancer diagnosis.
6. Methods for educating current workers
Investing in your present employees' AI and ML abilities is a must. Put in place training programs to improve staff members' skills, with an emphasis on cutting-edge AI tools and methods. As a result, the team's performance will improve, and your company will be able to keep AI/ML projects running smoothly and come up with new ideas without having to depend so much on outside help.
Drawing from successful examples and industry best practices, including these factors in your AI/ML project implementation plan will provide a solid groundwork for success.
Digital transformation challenges and how to overcome them
Strong post-implementation methods that boost operational efficiency and reduce risks are becoming more important as more and more firms use generative AI technology. Several essential components make a thorough strategy:
Check if your key performance indicators (KPIs) are in line with your organization's objectives and evaluate them regularly. Companies that consistently adjust their AI models to match their long-term objectives see a 25% increase in effect.
For instance, KPI and analytics help Netflix make better business choices and provide better customer service. A key component of Netflix's strategy to attract and keep users is its content recommendation engine, which has been upgraded with the help of analytics and artificial intelligence. Netflix regularly tweaks its recommendation algorithms to make them more accurate and relevant based on user input and performance data.
Key KPIs to monitor:
Algorithm accuracy
User engagement
Product popularity
Demographic and behavioral trends
System performance
User feedback
Conversion rate
Click-through rate (CTR)
Response time
Error rate
By regularly monitoring these KPIs, businesses can ensure that their AI systems are optimized for maximum effectiveness and customer satisfaction.
How to measure the success of your digital transformation efforts?
AI systems are managed effectively and in compliance, continuous monitoring and frequent audits are crucial. A brief explanation with supporting data and examples from the actual world is this:
Organizations can keep tabs on the functioning of their AI systems in real-time with continuous monitoring, which helps to spot problems before they escalate. According to data compiled by Deloitte, businesses that use continuous monitoring are 60% more likely to identify and fix operational errors promptly, cutting down on downtime and operational risk.
One application case is in online retail, where the efficacy of recommendation algorithms is constantly monitored by e-commerce platforms. To keep its suggestions current and effective, the system detects changes in consumer purchasing habits and automatically updates them.
To be precise it monitors:
User engagement: Tracks metrics that indicate user interaction with the recommended products, such as: Click-through rate (CTR) and Conversion rate.
Product popularity: Monitors the popularity of recommended products, such as: Views, and Sales.
Demographic and behavioral trends: Keeps an eye on demographic and behavioral trends, such as: User demographics (e.g., age, gender, location); User behavior (e.g., browsing history, search queries).
User feedback: Collects user feedback on the recommendations, such as: User ratings and reviews of recommended products; User complaints or dissatisfaction with the recommendations.
By monitoring this data, the business owner can identify areas for improvement, optimize the recommendation algorithm, and ensure that it continues to provide relevant and effective suggestions to customers.
To uphold ethical AI standards and comply with ever-changing legal requirements, it is essential to conduct audits regularly. Preventing compliance difficulties and maintaining public confidence are both helped by these audits. According to a KPMG poll, the majority of IT executives (73%) think that frequent audits are very important for keeping AI systems secure and intact.
An example from the banking industry is the quarterly audits that are conducted to guarantee compliance with the Fair Credit Reporting Act (FCRA) for AI systems that are used for credit scoring. To maintain an accurate and fair credit scoring system, these audits seek algorithmic biases.
Consistent audits and constant monitoring provide a strong foundation that guarantees AI systems are operating within the law and ethical guidelines, while simultaneously improving their performance and dependability. In sectors where AI is having a major influence on operational choices and regulatory obligations, this preventative measure is crucial.
Regular upkeep keeps AI systems running smoothly and using the most recent data and algorithms. To keep the technology running smoothly and for as long as possible, this is essential. Regular maintenance may extend the lifetime of technology by 20% and minimize system downtime by up to 35%, according to statistical research from IBM.
The healthcare industry is a good example of an industry that regularly updates its AI systems to take into account new medical research and clinical guidelines when making a patient diagnosis. To better diagnose disorders from medical imaging, for example, an AI system used in radiology is regularly upgraded. Improving diagnosis accuracy by 15% via regular software upgrades and algorithm tweaks has a direct influence on patient outcomes.
In the automotive industry, for instance, regular software maintenance is essential for autonomous driving systems. These upgrades strengthen decision-making algorithms using the most recently collected driving data and fix vulnerabilities that have just been found. For instance, Tesla's Autopilot software undergoes frequent upgrades that improve functionality and safety, taking into account user input and real-world driving data.
Consistent upkeep prevents operational and reputational disasters by keeping AI systems running smoothly and preventing them from malfunctioning. Industries whose technology has a direct influence on day-to-day operations and long-term strategy must adopt this proactive approach.
To keep AI/ML models accurate and relevant over time, it is vital to keep them updated. Models can adjust to new data and shifting conditions with regular updates. To combat emerging fraud trends, financial institutions regularly upgrade their AI fraud detection algorithms. As an example, a bank that consistently upgrades its algorithms for detecting fraud was able to substantially safeguard its clients' funds by reducing fraud instances by 20%.
The effective integration of AI systems depends on the organization's change management strategies. As part of this process, the organization must be ready for change, the transition must be managed, and the change must be solidified. Projects with robust change management methods had a 70% better chance of reaching their goals, according to Gartner research.
One of the most important parts of digital transformation is dealing with employee opposition to AI and ML implementations. Clear and straightforward communication that links the organization's objectives with the cross-functional team and professional advantages for workers is essential for effective transition management. The key to encouraging excitement and acceptance is making sure everyone knows how these technologies may improve efficiency and provide new possibilities.
Staff are empowered to respond to changes with confidence when they are given the required assistance via education and tools.
To ensure staff buy-in and minimal opposition to the new technical framework, it is critical to show that you are committed to their development and success inside it.
Through careful attention to these details, businesses will be better equipped to handle obstacles that arise after the installation of AI and ML, allowing for a more seamless integration into their digital transformation initiatives.
How the Mayo Clinic and Google Cloud collaborated to get staff on board with AI and ML deployments is an inspiring real-world example. The two organizations' shared goal was to improve healthcare operations and clinical procedures via the use of artificial intelligence and machine learning.
A software-driven strategy is quickly giving way to an AI/ML-driven one in the corporate world. Machine learning and artificial intelligence have several real-world applications across all industries.
To recap, key points of a successful strategy for digital transformation with AI/ML:
Set and track key performance indicators
Conduct continuous oversight and auditing
Maintain systems regularly
Update AI/ML models regularly
Implement organizational change management strategies
Secure employee buy-in and address resistance
To assist your company in taking the correct and strategic technological step toward improved digital transformation, Devico provides first-rate generative AI services. Get in touch with the Devico specialists at our organization right away; we are the best at what we do!
Outsourcing
Apr 16th 25 - by Devico Team
10 burning questions for software outsourcing companies to answer before you decide which one will be your partner.
Outsourcing
Apr 15th 25 - by Devico Team
Align your in-house and outsource software developing teams for seamless cooperation and better project outcomes.
Outsourcing
Apr 8th 25 - by Devico Team
Learn the benefits of implementing Agile in outsourcing and how it will change your project.