Staff augmentation
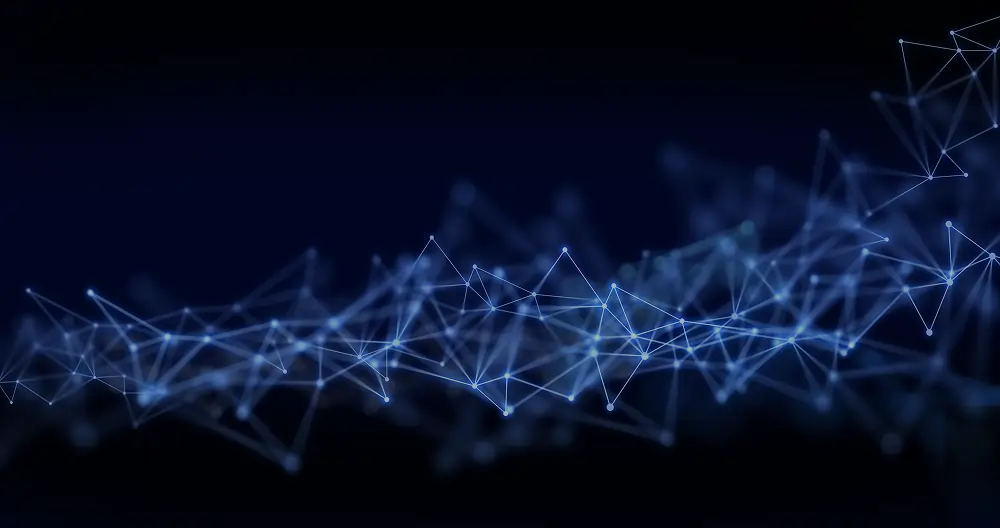
Outsourced developers vs freelancers: Which is better in 2025?
Feb 18th 25 - by Devico Team
Explore a detailed comparison of the differences, pros, and cons of partnering with outsourcing companies vs freelance for your project.
Data engineering
July 03, 2024 - by Devico Team
Data-driven decision-making is a new trending business approach that opens up new capabilities for growth and development. All industry leaders are already two steps ahead by using data to improve their key services. Amazon uses data to build targeted marketing campaigns based on customers' location and buying behavior. While Netflix customizes suggestions, minimizes customer churn, and boosts retention rates by analyzing customers' data. So, transforming your business into a data-driven organization is a must to get a competitive advantage and success in achieving your business goals.
However, only 13% of organizations are currently delivering on their data strategy, according to an MIT Tech Review and Databricks study. Such a small number of companies that have succeeded in working with data points to challenges, issues, and bottlenecks that slow down businesses or lead them to failure. Still, a smart approach to drawing up a data governance plan to optimize your business and get the most out of your data can help you avoid most obstacles.
So, what is data strategy, and why should you even care? As companies face an ever-increasing amount of data, it's critical to have a clear, comprehensive plan that describes how to collect, analyze, and use data to make informed decisions. In short, these are common elements of well-structured data planning. It helps businesses identify operational gaps, improve customer engagement, and boost growth. Moreover, robust data strategies can back risk management by providing information about the data collection types, sources, access permissions, and storage methods. This insight is critical to identifying potential vulnerabilities and preventing data breaches.
While you'll hardly find a unified data governance plan that fits everyone, there are several common mistakes you should avoid when creating one for your organization. In this article, we'll explore them and try to provide a solid solution to each issue.
The implementation of data strategies is beneficial for companies for many reasons. They help the planned implementation of technologies, allow you to make accurate and well-founded decisions, and identify new opportunities for business development. However, in the pursuit of advantages and in attempts to overtake competitors, be sure to pay attention to the following points.
Data strategies often fail when they are not aligned with specific business goals, which ends up in activities that do not support the overall business strategy. For example, a company may invest in big data technologies without having a clear plan for using the collected data. As a result, it wastes valuable people and budget resources.
Enthusiasm about the potential of data without sufficient strategic planning or understanding of business needs is often the cause of this mistake.
“Building a successful data strategy at scale goes beyond collecting and analyzing data,” says Ryan Swann, chief data analytics officer at financial services firm Vanguard. “Without mission orientation, it can be especially challenging for organizations to prioritize data initiatives, allocate resources efficiently, and foster an engaged workforce that has a clear understanding of how their work has a genuine impact on business outcomes.”
Step-by-step solution:
Involve key stakeholders in defining clear business goals.
Align each data initiative with these goals, using them as a basis for planning and execution.
Regularly review and adjust the data strategy to ensure it meets business objectives.
Aligning your data management actions with business goals can lead to improved decision-making efficiency. As a result, you'll get greater ROI from data projects, and make a way to achieving main ambitions, such as increased market share or improved customer retention.
Companies can rush to implement the latest data processing technology without an underlying strategy. For example, purchasing an advanced analytics platform without a usage plan will lead to underutilization. Too much faith in a technology that still cannot act on its own and "smart" can lead you to a merry dance. Another mistake, choosing technologies without taking into account further growth and development, or looking too far into the future: spending on data strategy technology that is not needed at the moment (and for a long time to come) will empty the budget too quickly.
This mistake can arise from the misconception that only technology can solve business problems. Another reason is the pressure to keep up with industry trends.
Step-by-step solution:
Prioritize strategy development before making any technology decisions.
Be clear about what business problems you are trying to solve and choose technologies based on them.
Make sure employees understand how to effectively use technology as part of the strategy.
By focusing on strategy before investing in technology, companies avoid costly mistakes and ensure that the technology they deploy is the best fit for their specific needs. Starbucks implemented its Mobile Order & Pay app looking for a specific solution for their main issues, like, long wait times, order accuracy, personalization orders, and payment options. Putting strategy in the first place, they've managed to create solutions that helped them resolve capacity issues and significantly boost sales.
Let's be honest, information only benefits the company if it is of high quality, cleaned, and prepared for analysis. Inaccurate, incomplete, or out-of-date data can mislead decision-making processes. For example, making marketing decisions based on data that has not been cleaned or updated leads to poor customer targeting. According to the survey, only 37 percent of respondents said that their efforts to improve data quality had been successful.
Inadequate data collection methods, lack of ongoing data management, or lack of attention to it are among the main reasons for this error.
Step-by-step solution:
Implement rigorous data management practices that include regular data quality reviews, and validation rules.
Apply reliable data-cleaning tools and procedures.
Assign data governance roles to ensure accountability.
By investing in data best practices, companies can improve the accuracy of their forecasts, improve customer analytics, and reduce the risk of costly errors. Overall, this can lead to better customer experience, more focused marketing strategies and more efficient operations.
Storing data in isolated systems can hinder comprehensive analysis. For example, customer information held separately by marketing and sales departments can lead to inconsistent customer acquisition strategies. When different departments, divisions, or groups keep data stored in systems inaccessible to others, it reduces the value of the data.
"Data silos result in inconsistencies and operational inefficiencies", says John Williams, executive director of enterprise data and advanced analytics at RaceTrac.
Data silos often evolve naturally as different departments and systems implement their own solutions without a coordinated approach.
Step-by-step solution:
Develop a centralized company-wide data integration framework.
Use integration tools such as ETL (Extract, Transform, Load) systems and APIs to facilitate data flow between systems.
Being one of the biggest retail pharmacy companies in the USA, Walgreens had an issue with data silos that had to be solved promptly. By adopting the new centralized Data Intelligence Platform they were able to break down these silos and gained a 360-degree view of the customer journey. Making more informative decisions they enhanced personalized marketing and improved sales figures.
A lot of data is collected every day. Its analysis usually takes much longer, due to which there may be delays in making urgent decisions on time. It also narrows the perspective and vision of the company, which affects the construction of the general strategy for growth and development. Moreover, a company that is unable to use real-time data can lose the capability to adapt to changes in customer demand and provide a better customer experience.
This is usually caused by the use of legacy data systems that do not support real-time processing or the lack of implementation of modern technologies.
Step-by-step solution:
Invest in real-time data processing tools and dashboards that enable instant analysis of business operations.
Train employees on how to interpret and act on real-time data.
Artificial or natural restrictions on access to data can prevent broader data-driven decision-making. For example, if only senior management has access to performance data, lower-level managers may make less informed decisions. When developing strategic data management and configuring access controls, you must consider all stakeholders according to their roles.
Usually, the main reason for limited access to data is data security concerns or a hierarchical company culture. In addition, decentralized data storage also leads to limited access for different departments and groups.
Step-by-step solution:
Implement role-based access control to ensure data security and expand access.
Promote a culture of informed decision-making at all levels of the organization.
Here is how Spotify benefited, having a more scalable and usable data approach. By transforming its data platform, Spotify democratized access to data among its teams, including data scientists, engineers, and product and business teams. It empowered them to make data-driven decisions to increase campaign effectiveness and overall user engagement with personalized music recommendations.
Learn how to drive maximum business value from big data development
It may sound obvious, but neglecting data protection laws, like GDPR, can lead to legal sanctions and reputational loss. Moreover, the protection of data (customer and intra-corporate) is an undeniable responsibility of the company. That is why it is crucial to implement modern protection methods, such as firewalls, security protocols, and encryption. Also, the human factor is still one of the main reasons for the majority of successful cyber attacks, hence, you have to constantly work with personnel.
This error comes from insufficient knowledge of the law or an underestimation of the compliance importance. Also, neglecting data protection rules can play a bad joke on the company.
Step-by-step solution:
Regularly train all employees on data privacy and security requirements.
Review and update data processing policies and practices to comply with the latest regulations.
Automate or implement convenient and reliable security and compliance testing tools.
While developing a comprehensive data strategy companies often allocate considerable investments in both technology and personnel. According to the survey, conducted on Gartner Data & Analytics Summit, 56% of data leaders said they increased their budget for D&A in 2023. However, misjudging the scope and scale of data initiatives, such as starting a large data integration project without sufficient budget for the necessary infrastructure, software, and skilled personnel, can lead to significant budget overruns and under-resources. As a result, this underestimation affects not only the current project but also its long-term sustainability and scalability.
This error often comes from insufficient or hasty planning, failure to account for hidden costs (such as maintenance and training), and overly optimistic assumptions about performance improvements and resource needs. Organizations may also overlook the need for specialized expertise or ignore the complexities associated with data migration and integration.
The UK's NHS (National Health Service) can be a great example of IT failure due to underestimation of costs and resource requirements. They were trying to create an electronic health record system and predicted costs of £2.3 billion. Over the next few years, the cost of the project rose to over £10 billion before it was finally abandoned.
Step-by-step solution:
Conduct detailed planning and feasibility studies before launching data projects.
Accurately define data strategy goals and forecast the resources needed at different stages of the project.
Include contingency funds in budgets and make sure you have a commitment to the necessary resources throughout the project.
With a reasonable approach to troubleshooting, you can combine a few solutions to enhance results. For instance, you can apply comprehensive tools and practices for data gathering and cleaning to avoid data silos and level up its quality.
When building a data strategy, always consider the specifics of your company, because industry or technology trends are not always the appropriate solution for peculiar tasks and needs. Also, be careful with the introduction of new methodologies, tools, and ways of working with data — take your time. Sometimes it is worth spending more time on preparation and getting a reliable, not a quick result.
Data planning aims to streamline the ways and methods of collecting, processing, analyzing, and storing data to get the most out of them. However, building a reliable and effective data management strategy is accompanied by a number of challenges and mistakes that should and can be avoided. Trying to outrun your competitors, you can slow down or even lose if you don't check your data plan in time.
By following these guidelines and continuously improving your data planning, you can find gaps in it, or, if you're at the very beginning of the way, avoid them. Adopt best practices and solutions to unlock the true potential of your data and gain a competitive edge. Although they say that the best is the enemy of the good, leave room to scale your plan. This will make it possible to make timely changes and create a really working and effective business data approach.
Staff augmentation
Feb 18th 25 - by Devico Team
Explore a detailed comparison of the differences, pros, and cons of partnering with outsourcing companies vs freelance for your project.
Staff augmentation
Feb 13th 25 - by Devico Team
Having offshore teams in various time-zones is an invisible advantage for companies growth and productivity in the software development industry.
Staff augmentation
Feb 11th 25 - by Devico Team
Hiring offshore developers can be a game-changer for your startup. But is it worth it in the long game?